AMiner × Qubit Co-Production
Editor's note:
Like many western cities, Chongqing is often criticized as an "Internet desert", but it has a good soil for the Internet of Things.
According to statistics, Chongqing has nearly 2,000 IoT-related companies, of which more than 600 are core companies, and they are strong and influential in the IoT industry segment.
In addition to enterprises, Chongqing also has a special Internet of Things technology "power": the Internet of Things Somatosensory Big Data Laboratory of Chongqing University, led by Liu Li.
Liu Li is currently a professor and doctoral supervisor at the School of Big Data and Software, Chongqing University.
And he established this laboratory in 2018, hoping to build a bridge between causal learning and machine learning.
This direction is also an area where big bulls such as Bengio and Pearl are also focusing on investment.
From a more specific application point of view, Liu Li believes that after this goal is achieved, AI and big data analysis technology can have greater use in medical, education, manufacturing and other fields.
We had the pleasure of having an in-depth conversation with him.
A typical scholar's "three steps"
For Liu Li, there has always been a clear direction guiding his career growth, which is to combine what he has learned with major practical issues at the national level .
In particular, in recent years, the country has launched several major measures for big data, artificial intelligence, industrial Internet, etc., hoping to realize the deep interconnection of people, machines and things, and realize the connection of all elements and the entire value chain, and then promote new innovations. Industrial manufacturing and new service systems.
Chongqing is an old-fashioned industrial city. Therefore, Liu Li returned to China and led the establishment of the IoT Somatosensory Big Data Laboratory at Chongqing University, aiming to carry out basic research and application of big data analysis, especially causal learning, around the country's major needs.
In fact, before establishing the IoT Somatosensory Big Data Lab, he has been dealing with big data for decades.
At the undergraduate and master's level, Liu Li studied under Professor Li Lian of Lanzhou University .
Professor Li Lian is a leader in computer education in China. He has long insisted on promoting the concept and new model of computer talents with computational thinking as the core. For this reason, he won the CCF Outstanding Education Award in 2020.
Influenced by him, Liu Li participated in the China Education and Research Grid Project in the second half of the undergraduate and master's stages. The grid can be regarded as the predecessor of the cloud platform to solve the problem of data storage and processing.
This experience established his way of thinking: a computational thinking-led approach to problem-solving around the storage and processing of data .
But he truly established his scientific research dream when he was studying in a foreign country.
In 2007, when Liu Li was studying for a Ph.D. at the University of Paris 11, the Nobel Prize in Physics was awarded to Gilles Albert Fehr, a professor at the University of Paris 11. At that time, the whole school was boiling. This news inspired the teachers and students of the whole school, including Liu Li. .
Liu Li said:
This made me more determined to choose the academic path.
At that time, the prestigious Paris Eleven University had already won 2 Nobel Prizes and 4 Fields Medals. The school advocates a scientific problem-oriented approach to basic research.
More importantly, these problems are not necessarily all from the application direction, but mostly from the basic direction.
At the same time, the overall relaxed and free environment in France allows him more time to think independently about the differences between basic research and technology applications. Liu Li recalled:
In spring, France has various holidays. In summer, everyone, regardless of occupation, will go on vacation. In autumn, strikes start, and in winter, they start preparing for Christmas.
In this atmosphere, basking in the sun by the banks of the Seine, the time just passed. Sometimes our Chinese students want to go to the laboratory to work for a while at night, and the police will come to ask whether the rights and interests of overtime are guaranteed, and they cannot work overtime without special approval.
Under the influence of this atmosphere, we also calmed down and thought about how we should do basic research.
After that, Liu Li has completed the establishment of computational thinking and the recognition of basic research. But he found a real world of his own on a new coordinate.
Farewell to the romantic and leisurely Paris, in the tropical country of Singapore, Liu Li and Academician David S. Rosenblum, Dean of Computing at the National University of Singapore, jointly launched a research called " Felicitous Computing " (Felicitous Computing) .
The essence of this concept is to adjust the computing power according to different scenarios.
At this point, computing has become richer than when Liu Li first came into contact with big data analysis. It not only extends to everywhere, but also presents the characteristics of the ternary integration of people, machines and things centered on people.
How to deal with these new big fusion big data has become the most concerned issue of Liu Li and his colleagues.
During this period, he and his team also made a lot of representative research, such as From action to activity: sensor-based activity recognition.
They innovatively correlated human short-term action data with activity data in a wider space-time dimension , bridged the gap between human actions and human activities, and successfully encoded the gap between actions. temporal correlations and capture the intrinsic properties of activities.
It was also at this stage that Liu Li really established his research direction, which is to collect and analyze data around the physical world and people themselves.
From the establishment of computational thinking, the recognition of basic research to the establishment of specific directions, the diversified experience during this period has also given him a more three-dimensional perspective and feeling on big data and even data science.
This is why the IoT Somatosensory Big Data Lab of Chongqing University has such a concrete name.
Liu Li believes that to understand big data analysis from an application perspective , it can be regarded as a car :
There must be a lot of components in this car to support big data analysis. The engine is the computing power platform such as CPU and GPU; after having the computing power, if you want to run, you must have oil, which is data; and the wheels are like applications, that is, the bottom car Where to go, what goal to move towards.
To achieve these applications and achieve goals, you need to have a steering wheel, which is to use algorithms to direct all these things.
In his words, the work he has done over the years involves both engines, oil, wheels, and steering wheels, including the underlying framework for writing a lot of data processing in the early days.
But in this new laboratory, naturally it will not be all the research that I have experienced and familiar with. There are still many things to do from scratch, and there is even a lack of DIY gloves for IoT research with students. and watch.
For the entire team, Yuan Fang has a bigger goal, which is to build a bridge between causal learning and machine learning.
The three major "difficulties" of machine learning, causal learning is the breakthrough
At present, machine learning and big data have developed to a stage where you are in me and you are in me .
In particular, machine learning represented by deep learning has made good progress. At the same time, the "defects" of such methods have become increasingly prominent.
In particular, these main issues: interpretability , generalizability , robustness .
How can the solution of these three problems be promoted at the same time?
A promising solution is to introduce causal learning into machine learning, which can promote the interpretability of machine learning methods, improve stability, and generalize to different scenarios.
Regardless of deep neural networks or some other methods, most of the learning is the correlation between variables and variables, ignoring the causality between variables. The latter is the focus of causal learning.
For example, in cancer prediction, causality is very important, which attributes/variables lead to the occurrence of cancer outcomes is an explanatory question that both doctors and patients want to know.
For a long time, machine learning and causal learning were two relatively independent research directions, but now, this situation has changed, and more and more artificial intelligence scholars are aware of the potential of causal learning.
One of the representative figures is Bengio, one of the "Big Three of Deep Learning".
He has stated:
Integrating causality into AI has become a top priority right now. Current machine learning implementations are based on the basic assumption that when a trained AI system solves a practical problem, the data it faces is of the same type as the training data. But in real life, the situation cannot be so simple.
Also in recent years, he led a team into this new research focus: causal representation learning that combines machine learning with causal inference.
Another important force is Schölkopf and his team at the Max Planck Institute, who are also exploring how to create AI systems that can learn causal representations.
Liu Li and his team also see "building a bridge between their causal learning and machine learning" as a long-term mission.
In addition to basic research, they can also rely on the industrial soil of Chongqing, so that the causal framework theory can be implemented.
For example, in image synthesis, the emergence of GAN and VAE has allowed people to see the shocking effect of image synthesis. However, these generation capabilities are still stretched in some scenarios that emphasize controllable image generation.
Like car modeling generation, it is very different from artistic image generation, and must meet certain industrial standards, so when computer-aided modeling is generated, it must be controllable.
Controllability is a difficult problem to solve at present. This scenario is also the focus of Liu Li's laboratory to explore, that is, to use the causal method, starting from the bottom layer of the data generation mechanism, to discover the correlation of data, and to make features at the level of correlation Decoupling, to learn what factors ultimately lead to underlying problems such as tire size changes.
In the next few years, the application directions of image synthesis, disease diagnosis and behavior recognition will be their key research directions.
To roll or not to roll? Advice for young students
During the exchange, when talking about his way of studying and seeking knowledge, Liu Li always mentioned, "combining personal goals with the major needs of the country".
This is not only his request for himself, but also his hope for young students. Now the whole field of computer science is discussing the phenomenon of "involution". He especially hopes that his experience and experience can help some young students "break the problem":
"First of all, we must pay attention to basic theories and make them solid. At present, learning data science and machine learning is particularly modular. It is possible to find an algorithm on the Internet and then assemble it to solve a task. This application-oriented preference is in It is understandable to quickly solve problems in the workplace, but from academia or from the lowest level of logic, this phenomenon is not necessarily a good thing.
This may be caused by the fact that our young people have to produce something quickly because they are too introverted. This phenomenon exists not only for undergraduates, but also for some doctoral and postgraduate students.
Now causal learning is very hot, maybe after some causal concepts are mixed into the neural network to have some effect, you can also post a very good article. It's not to say that you can't do this, but after completing this step, can you think about what kind of core problem you have solved?
This is also my second suggestion. Think about what the country's major needs are, including economic development, social governance, and people's livelihood, etc. What does my work promote in these areas? How much has he contributed to the problem of stuck necks and leading issues?
Nowadays, young people are very powerful. They can send good top-level articles and solve some problems quickly. But what kind of major needs do these methods and models solve?
I think that young people may need to think about these issues from time to time, whether it is to start a business or continue to stay in the academic circle, they should ask the core questions from a higher perspective and examine what they have done. "
About Liu Li
Liu Li, professor and doctoral supervisor of the School of Big Data and Software, Chongqing University, founded the Internet of Things Somatosensory Big Data Laboratory of Chongqing University.
He has been committed to the research on sensing big data analysis technology and its application, and presided over more than 10 general projects of the National Fund Committee, youth funds, sub-projects of the National Major R&D Program, major special projects of the Chongqing Municipal Science and Technology Commission, and the Ministry of Education's scientific research start-up fund. item.
At present, Liu Li has published more than 100 papers and applied for more than 10 invention patents.
At the same time, he also serves as the guest editor of international SCI journals such as Sensors and Web Intelligence.
Served as the chairman and member of the program committee in CCF ABC international conferences or seminars such as UbiComp, CSCW, etc., as the secretary general of the National Wearable Computing Conference, and IEEE Transactions on Cybernetics, IEEE Intelligent Systems, IEEE Transactions on Learning Technologies and other top artificial intelligence journals 's review.
Homepage:
https://www.aminer.cn/profile/li-liu/56065cde45cedb3396854f9a
About the AI 2000 list:
In January this year, the AMiner team launched the AI 2000 list, which aims to select the most influential and dynamic top scholars in the field of artificial intelligence in the past ten years through AMiner academic data, and praise them for their contributions to the field of artificial intelligence research. Outstanding contribution.
Click [read the original text] to learn more about the complete content of the AI 2000 list.
-End- _ _
Download "AI Pharmaceutical In-depth Industry Report · Qubit Think Tank"
After interviewing dozens of institutions and conducting in-depth research, the qubit think tank wrote the "AI Pharmaceutical Industry Report" , which can be downloaded by scanning the QR code below.
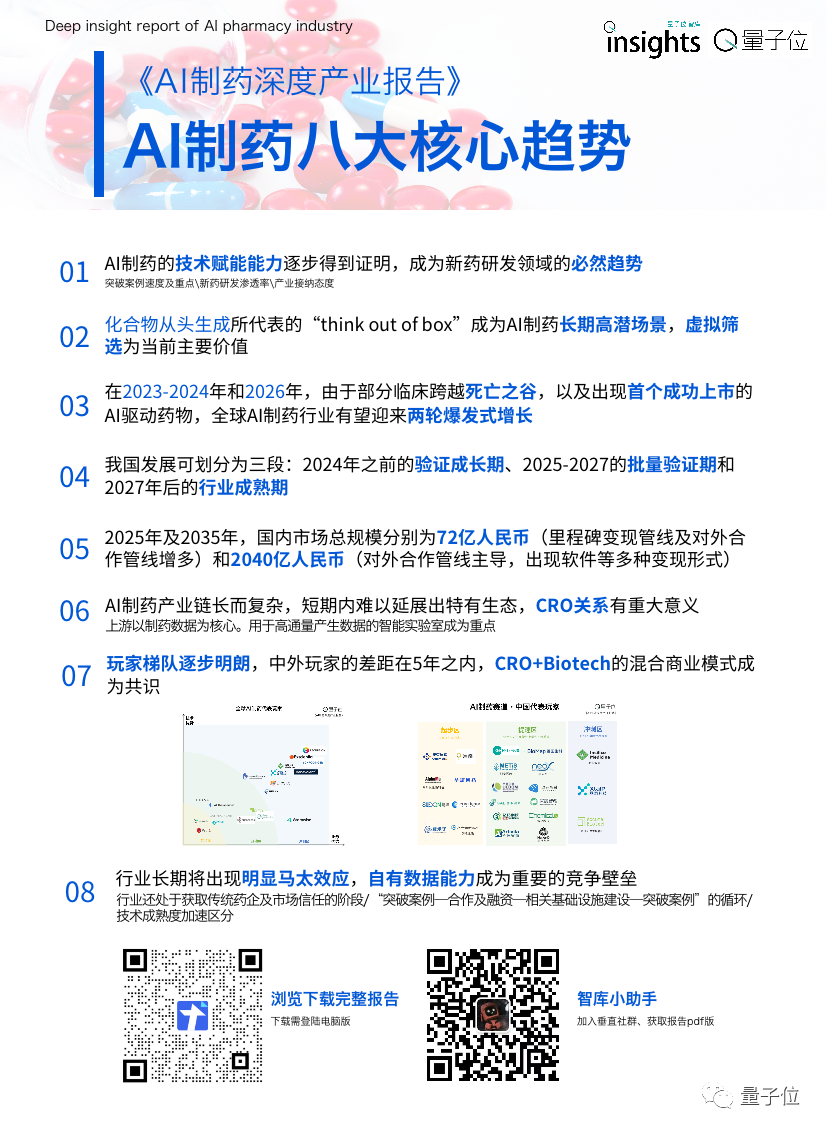
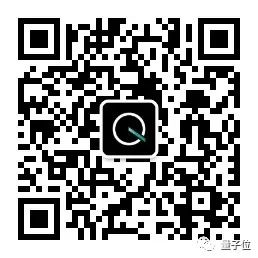
Qubit QbitAI · Toutiao signed author
վ'ᴗ' ի Track new developments in AI technology and products
一键三连「分享」「点赞」和「在看」
科技前沿进展日日相见 ~