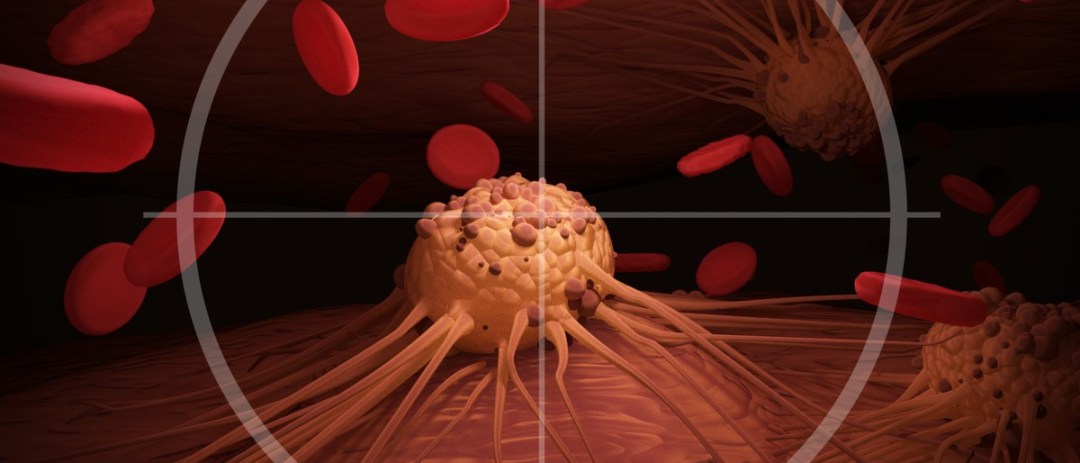
Xinzhiyuan report
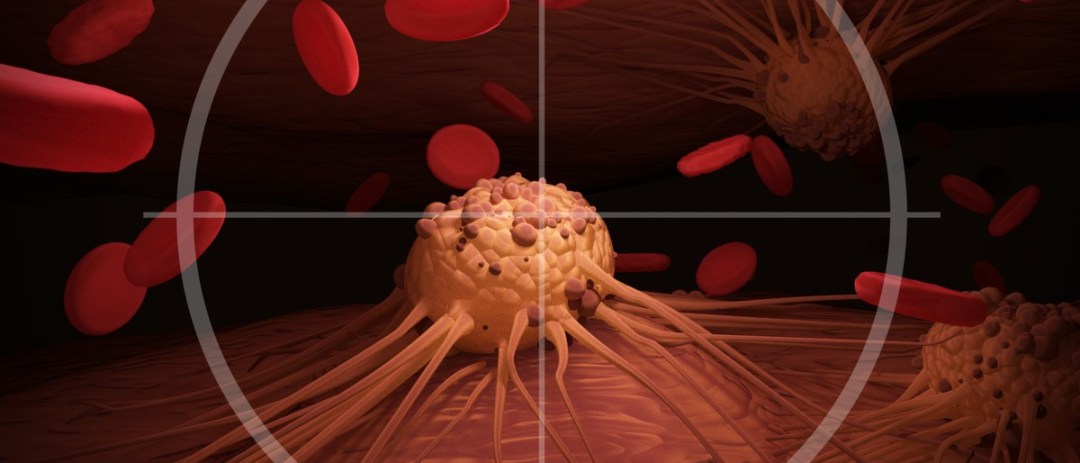
Xinzhiyuan report
[New Wisdom Introduction] Recently, a machine learning algorithm "Ikarus" developed by the team of MDC bioinformatician Altuna Akalin has deciphered the genetic characteristics of cancer cells with an accuracy rate of up to 99%.
AI has done another job.
This time, a new AI machine learning algorithm "Ikarus" can decipher the difference between the genetic characteristics of cancer cells and normal cells.
The research was completed by the team of MDC bioinformatician Altuna Akalin and published in the Nature sub-journal "Genome Biology".
Paper address: https://genomebiology.biomedcentral.com/articles/10.1186/s13059-022-02683-1#Sec8
In addition, the MDC (Max Delbrück center) responsible for this research is also one of the 16 research centers of the Helmholtz Association, one of the four major research institutions in Germany.
Since it is so big, why is this research so important?
A "common feature" is screened out from the vast data set, and humans are definitely not as good as AI.
To distinguish cancer cells from normal cells, it is necessary to screen out the common features between them.
This time, Ikarus, developed by the MDC research team, discovered a common pattern in tumor cells , which consists of a series of genomic features and is common in various types of cancer.
In addition, the algorithm detected gene types that had never been linked to cancer.
So the research team asked a simple question:
Is it possible to make a classifier that correctly distinguishes tumor cells from normal cells for multiple cancer types?
Thus, Ikarus was born. It consists of two steps:
1. Discover comprehensive tumor cell characteristics in the form of gene sets by integrating multiple professionally annotated single-cell datasets;
2. Train a robust logistic regression classifier to strictly discriminate between tumor and normal cells, and then use a customized cell-cell network for network-based propagation of cell labels.
Team leader Altuna Akalin said:
To develop a robust, sensitive and reproducible in silico tumor cell sorter, we have tested Ikarus on multiple single-cell datasets of various cancer types obtained using different sequencing techniques to determine its suitability for different experiments. surroundings.
amazing success rate
amazing success rate
Getting the right training data is a major challenge when experts already clearly distinguish healthy cells from cancer cells, said Jan Dohmen, the paper's first author.
Single-cell sequencing datasets are often complex.
This means that the information they contain about the molecular characteristics of individual cells is not very precise, because different numbers of genes are detected in each cell, or because samples are not always processed the same way.
Dohmen and study co-leader Dr Vedran Franke said,
We sifted through countless publications and contacted a considerable number of research groups to obtain adequate datasets. The team eventually selected data from lung and colorectal cancer cells to train the algorithm before applying it to datasets of other types of tumors.
During the training phase, Ikarus had to find a "list of signature genes," which was then used to classify cells.
We tried and improved various approaches, and Ikarus ended up using two lists: one for cancer genes and one for genes from other cells, Frank explained.
After training, the algorithm was able to distinguish between healthy and tumor cells in other types of cancer, such as tissue samples from patients with liver cancer or neuroblastoma.
In other samples, the results were exciting, with a surprisingly high success rate of up to 99%.
"We did not expect that there would be a common signature that defines tumor cells in different types of cancer with such precision," Akalin said.
"But we still can't say whether this approach works for all types of cancer," Dohmen added.
Not just cancer cells
To turn Ikarus into a reliable cancer diagnostic tool, the researchers now hope to test it on other types of tumors.
In initial tests, Ikarus has shown that the method can also distinguish other types (and certain subtypes) of cells from tumor cells, beyond just tumor cell detection .
It can be used to detect any cell state, such as cell type, the only requirement is that the cell state is present in at least two independent experiments.
Akalin said:
We hope to make this method more comprehensive and further develop it so that it can distinguish all possible cell types in biopsies.
Applying automated tumor classification on spatial sequencing datasets can directly annotate histological samples, thereby facilitating automated digital pathology.
In hospitals, pathologists often examine only tissue samples of tumors under a microscope to identify various cell types. This is a laborious and time-consuming job.
With Ikarus, this step could one day become a fully automated process.
In addition, Akalin noted, the data can be used to draw conclusions about the immediate environment of the tumor. This can help doctors choose the best treatment. The makeup of cancerous tissue and the microenvironment often indicates whether a certain treatment or drug is working.
In addition, artificial intelligence may also help in the development of new drugs.
"Ikarus allows us to identify genes that may contribute to cancer, and then new therapeutic agents can be used to target these molecular structures," Akalin said.
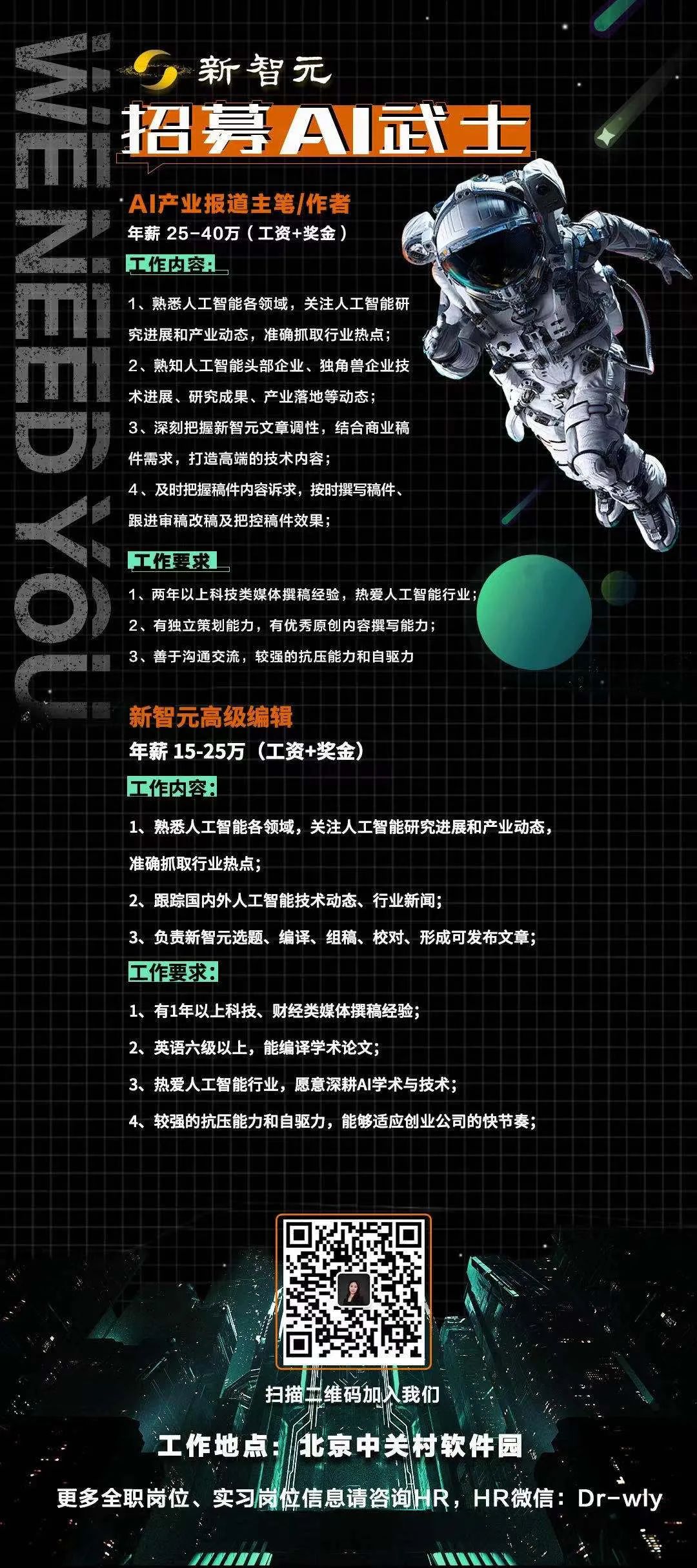
